Compound selection assisted by Bayesian inference of efficacy metrics at ISMB 2020
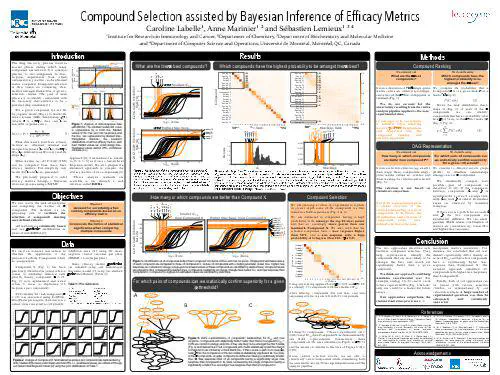
Caroline Labelle’s presentation: Compound selection assisted by Bayesian inference of efficacy metrics, has been accepted at the 28th International Conference on Intelligent Systems for Molecular Biology (ISMB) 2020. The poster is also published online.
Abstract
Chemical compounds are tested in various assays from which Efficacy Metrics (EM) can be estimated. Compounds are selected with the aim of identifying at least one sufficiently potent and efficient to go into preclinical testing. Selection is based on EM meeting a specific threshold or by comparison to other compounds. Current analysis methods only suggest estimates of EM and hardly consider the inevitable experimental noise, thus failing to quantify the uncertainty on EM on which conclusions are based. We propose to extend our previously introduced rigorous statistical methods (EM inference) to a panel of compounds. Given an efficacy criteria, we aim at identifying the compounds with the highest probability of meeting that criteria. We use a hierarchical Bayesian model to infer EM from dose-response assays. Given the empirical values distributions for an EM of interest, our novel ranking method returns the probability that each compounds within a set is able to achieve a given rank. We are able to identify all compounds of a experimental dose-response set with at least 1% chance of being amongst the best for a given EM. To further analysis, we generate DAGs where path between two compounds identifies which is statistically better.