Neural network development from pan-transcriptomic k-mer tables for RNA-Seq applications
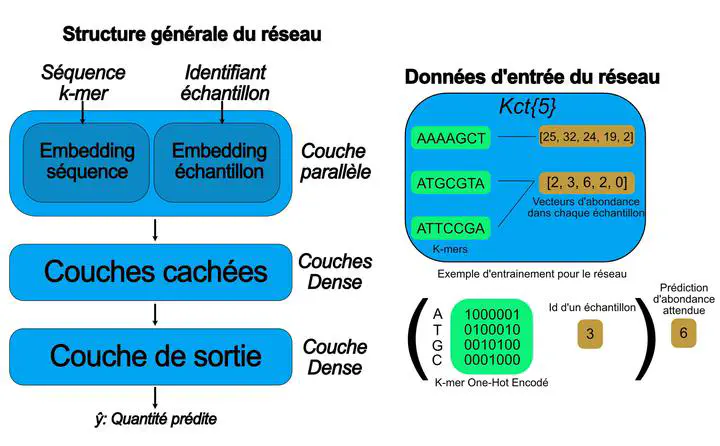
Although neural networks are capable of producing gene expression data embeddings, this type of data relies on an alignment of sequences with a reference, implying a loss of information with the sequences (even though expressed). not aligning with the reference. Training from RNA-Seq reads, divided into k-mers, however, involves a challenge linked to the dimensionality of the data. Although k-mers should be richer in information, it is difficult to extract due to the noise and dimensionality of this type of data. Generating a representative embedding of transcriptomic profiles from these data could not only have a predictive capacity at least equivalent to a classic profile, or even superior due to the richness of these data, but also would show that very short sequences contain sufficient information to the assembly of such a profile and would potentially allow sequencing with very short sequences.